The Ultimate Guide to Labeling Images for Object Detection
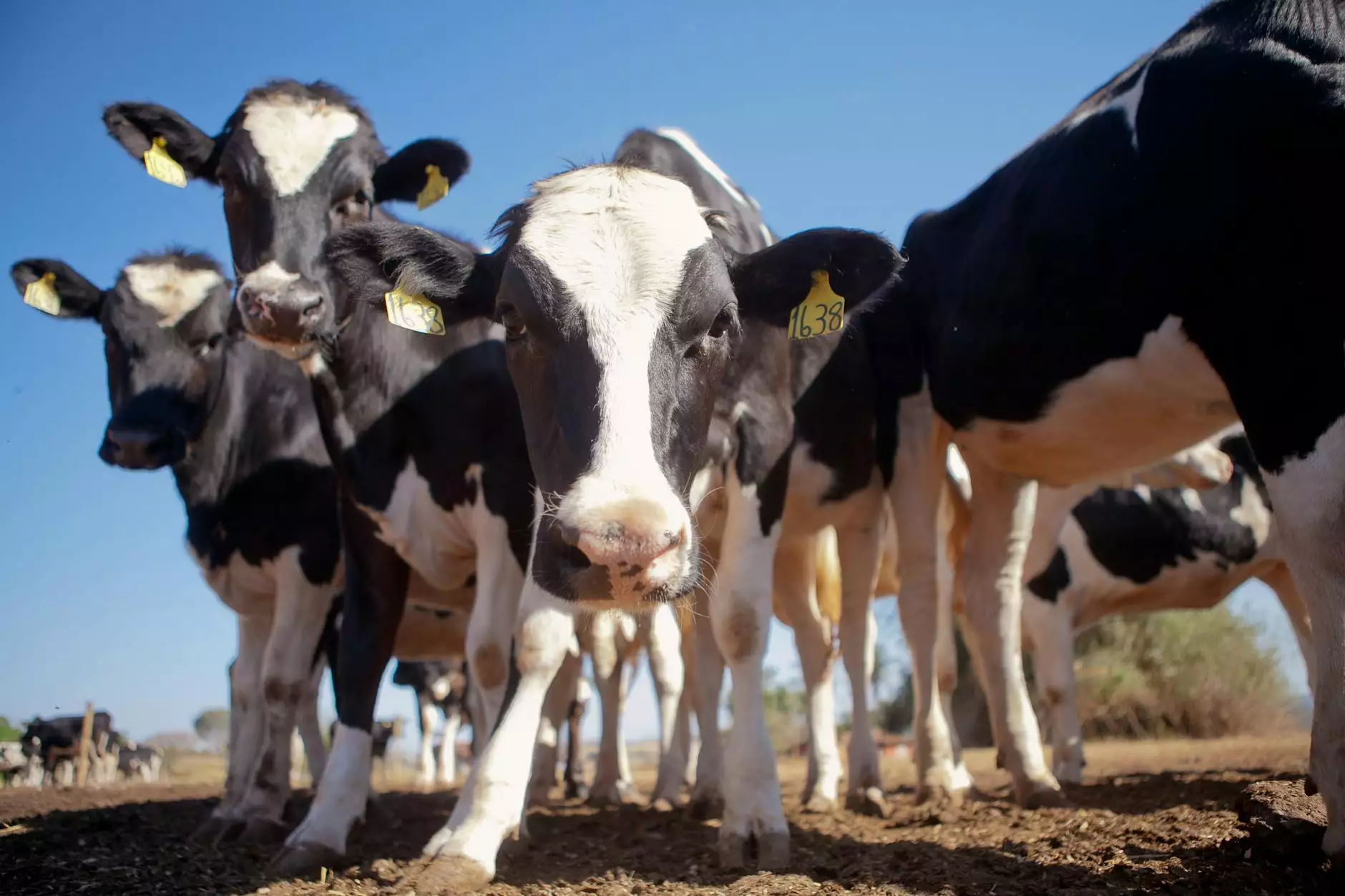
In today's rapidly evolving technological landscape, the demand for high-quality data annotation is at an all-time high, particularly in the field of object detection. Companies are leveraging advanced algorithms to automate processes and gain insights from data, and labeling images for object detection is a fundamental step in ensuring the success of these initiatives. This article, presented by Keylabs.ai, delves deep into the art and science of image labeling, highlighting the tools, strategies, and best practices that businesses should consider to thrive in this arena.
Understanding Object Detection
Before we dive into the specifics of labeling images for object detection, it’s essential to understand what object detection entails. Object detection is a computer vision task that involves identifying and locating objects within images or video frames. This technology is used in various applications, including autonomous vehicles, security surveillance, industrial automation, and more.
The Importance of Quality Data Annotation
Imagine training a sophisticated algorithm without accurate data; the outcome would be subpar at best. The quality of the data significantly influences the performance of machine learning models. For object detection systems, labeling images correctly ensures that models learn to recognize and interpret visual information accurately. Without precise annotations, the entire foundation of the system may crumble, leading to ineffective outcomes.
Types of Object Detection
There are primarily two types of object detection that are commonly discussed in the realm of machine learning:
- Single-Stage Detection: This method predicts bounding boxes and class probabilities directly from the image in one evaluation. Models such as YOLO (You Only Look Once) and SSD (Single Shot Detector) fall under this category.
- Two-Stage Detection: In this approach, the system first generates proposals (candidate bounding boxes) and then classifies those boxes. This process can be seen in models like Faster R-CNN.
The Role of Data Annotation Tools
When it comes to labeling images for object detection, the right tools can make a considerable difference in efficiency and accuracy. Keylabs.ai offers a user-friendly Data Annotation Tool designed to provide seamless integration of various labeling methods, ensuring that teams can focus more on accuracy rather than the intricacies of the software.
Key Features of Robust Data Annotation Tools
When selecting a data annotation tool, consider the following features:
- User-Friendly Interface: The tool should be intuitive, allowing users to navigate easily and complete annotations efficiently.
- Multiple Annotation Types: The ability to handle various types of data annotations such as bounding boxes, polygons, and semantic segmentation is essential.
- Collaboration Features: Tools that enable multiple users to work simultaneously can enhance productivity.
- Quality Assurance Mechanisms: Features that help assess the quality of annotations can reduce errors and improve the final output.
- Integration Capabilities: The ability to integrate with machine learning pipelines and data storage systems is crucial for streamlined workflows.
Effective Techniques for Labeling Images
Labeling images accurately is both an art and a science. Here are some techniques and best practices to consider:
1. Define Clear Annotation Guidelines
Establishing a set of clear guidelines is vital. Defining how to handle various scenarios, such as overlapping objects or partial visibility, can help maintain consistency across the dataset. Provide examples for your team to reference.
2. Utilize Pre-Annotation Features
Some advanced tools, including Keylabs.ai's Data Annotation Platform, provide pre-annotation capabilities that can automatically suggest labels based on existing data. This can save time and increase productivity by allowing annotators to verify and adjust proposed labels instead of starting from scratch.
3. Incorporate Quality Control Steps
Implementing a quality control step in the process can catch errors before they affect the training of machine learning models. This could involve peer reviews or using automated checks within the annotation tool.
4. Compile a Diverse Dataset
Having a diverse dataset is crucial for training robust object detection models. Ensure that your images encompass various scenarios, lighting conditions, and object variations to improve the generalizability of your models.
Challenges in Labeling Images for Object Detection
Despite improvements in tools and techniques, several challenges can arise when labeling images for object detection. Understanding these challenges is the first step toward mitigating them.
1. Subjectivity in Labeling
Due to the subjective nature of visual interpretation, different annotators may label the same image differently. Establishing strict guidelines and using multiple annotators can help reduce this issue.
2. Scalability Issues
As the volume of data increases, the time and resources required for data annotation can become overwhelming. Solutions such as outsourcing or employing automated annotation tools can aid in managing scalability effectively.
3. Dealing with Imbalanced Data
In many real-world applications, some objects may be overrepresented while others are underrepresented in the training dataset. Techniques such as data augmentation or synthetic data generation can help in alleviating this imbalance.
Best Practices for Data Annotation Teams
A well-structured data annotation team can significantly enhance the quality of your labeling efforts. Here are some best practices to follow:
- Continuous Training: Regularly train your annotators on best practices and guidelines to ensure ongoing quality improvement.
- Use Analytics to Monitor Performance: Implement analytics tools to track the efficiency and accuracy of your team, allowing you to identify and address any issues proactively.
- Encourage Open Communication: Fostering a culture of feedback can help improve processes and resolve ambiguities quickly.
- Implement Regular Audits: Conduct periodic reviews of the labeling process and outcomes to ensure standards are being met.
The Future of Image Labeling in Object Detection
As technology advances, the future of labeling images for object detection looks promising. Innovations in machine learning and AI are paving the way for smarter annotation tools that will minimize manual effort and enhance accuracy:
1. Artificial Intelligence and Machine Learning
AI-driven annotation tools can analyze image data to recognize patterns, automating much of the labeling process and allowing human annotators to focus on verification.
2. Synthetic Data Generation
Utilizing synthetic data to augment real-world datasets will allow for greater variability, enhancing model robustness without the costs associated with additional data collection.
3. Advanced Computer Vision Techniques
As computer vision technology evolves, the tools used for labeling images for object detection will become more sophisticated, allowing for more nuanced and complex annotations.
Conclusion
Labeling images for object detection is more than just a technical task; it is a vital part of the machine learning pipeline that can significantly influence the performance and success of your models. By investing in quality tools, employing best practices, and understanding the complexities of the process, businesses can harness the power of data to drive innovation and success.
At Keylabs.ai, we are dedicated to providing cutting-edge tools that simplify data annotation and ensure high-quality outputs. By following the guidelines and practices outlined in this article, organizations can enhance their image labeling efforts and achieve superior results in their object detection initiatives.
label images for object detection